2024 - 2025 Roberts Fund Awarded Projects
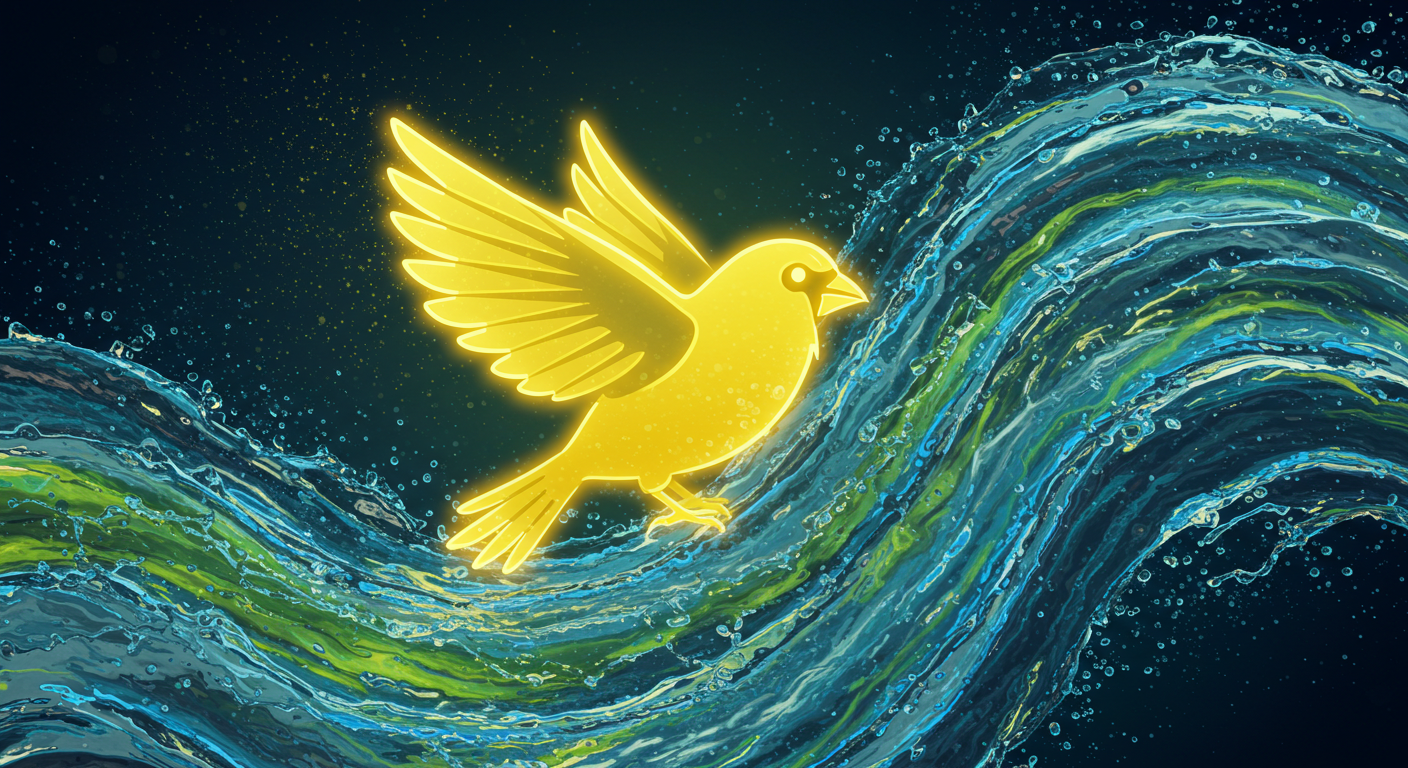
Canary sensor for household water purification systems
Project team
Jaehong Kim, Henry P. Becton Sr. Professor of Chemical & Environmental Engineering
Rilyn Todd, Ph.D. Candidate
Yonghyeon Kim, Postdoctoral Associate
The global market for household water purification systems is valued at $35B and continues to grow. Current systems lack sensors to monitor the quality of treated water. When these systems fail, harmful pollutants can be released and impact health. Their technology aims to help users know when the treated water contains pollutants. Just as miners in the past used canaries as air pollution sensors, by knowing to evacuate if a canary became ill, the team’s novel Canary Sensor will provide a similar warning for water quality. When the Sensor accumulates pollutants up to a predetermined level, it will warn the users about the system failure.
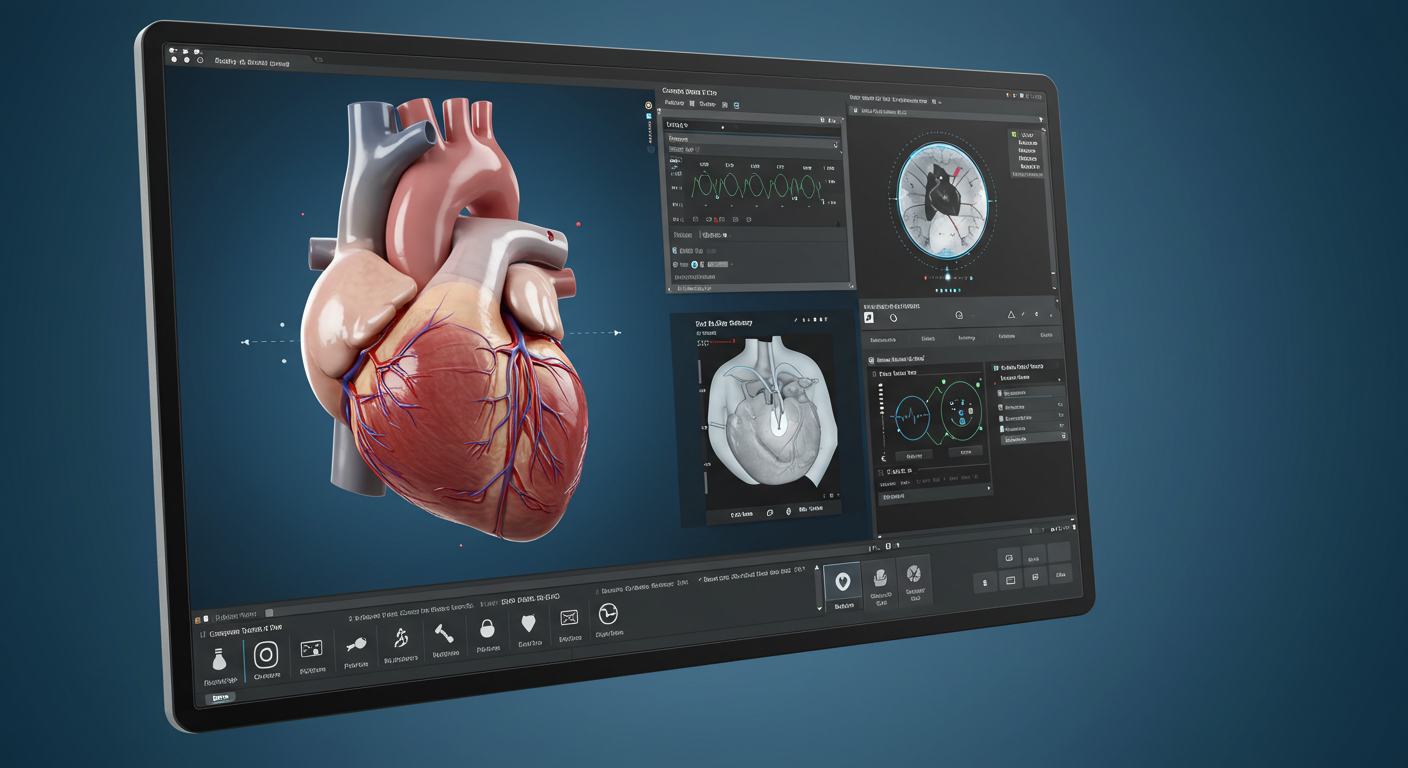
Carvis Health
Project team
James S. Duncan, Ebenezer K. Hunt Professor of Biomedical Engineering, Radiology & Biomedical Imaging, Electrical & Computer Engineering and Statistics & Data Science
Daniel Pak, Postdoctoral Fellow
Theodore Kim, Professor of Computer Science
The team has developed a software solution to provide scalable personalized simulations of heart surgeries, with the goal of decreasing complexity in surgical planning, and increasing the efficacy of the procedure. They intend to improve patient care, reduce complications by 50%, saving $2.9B in complication costs and decrease burn-out with minimal changes to overall workflow.
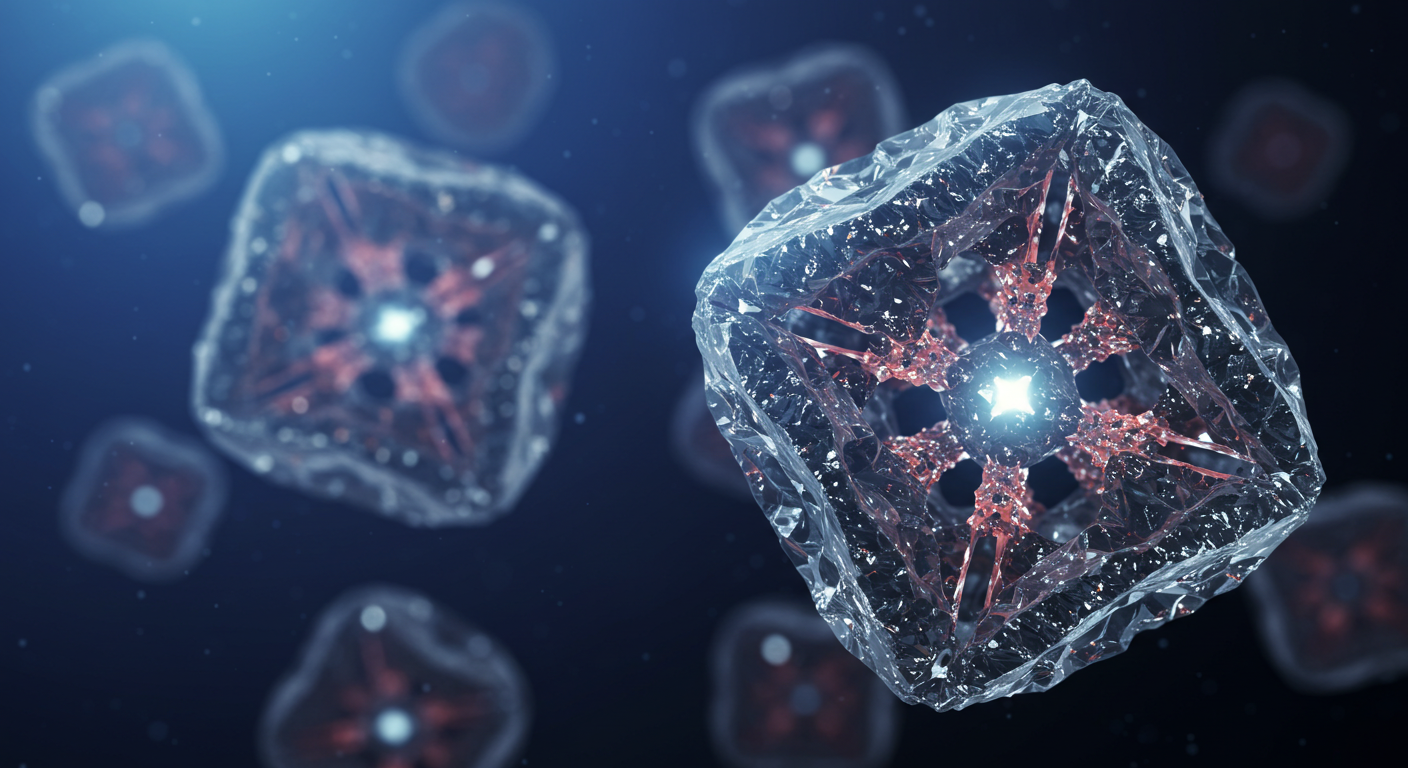
Compact ultra-high stability vacuum-gap reference cavities enabling portable precision metrology and sensing
Project team
Peter Rakich, Donna L. Dubinsky Professor of Applied Physics & Physics
Greg Luther, PhD, CEO and co-Founder of Resonance Micro Technologies Inc.
A wide range of technologies, including navigation (e.g. GPS), radar, sensing, and communications technologies rely on low-noise oscillators (i.e. clocks). The lower the noise of the oscillator, the more accurately users can identify the position of a vehicle, detect a microwave communications signal, or sense motion in a radar system. The Rakich Lab has invented first-of-a-kind ultra-high performance micro-resonators and oscillators that fill critical technology gaps in both classical and quantum application spaces.
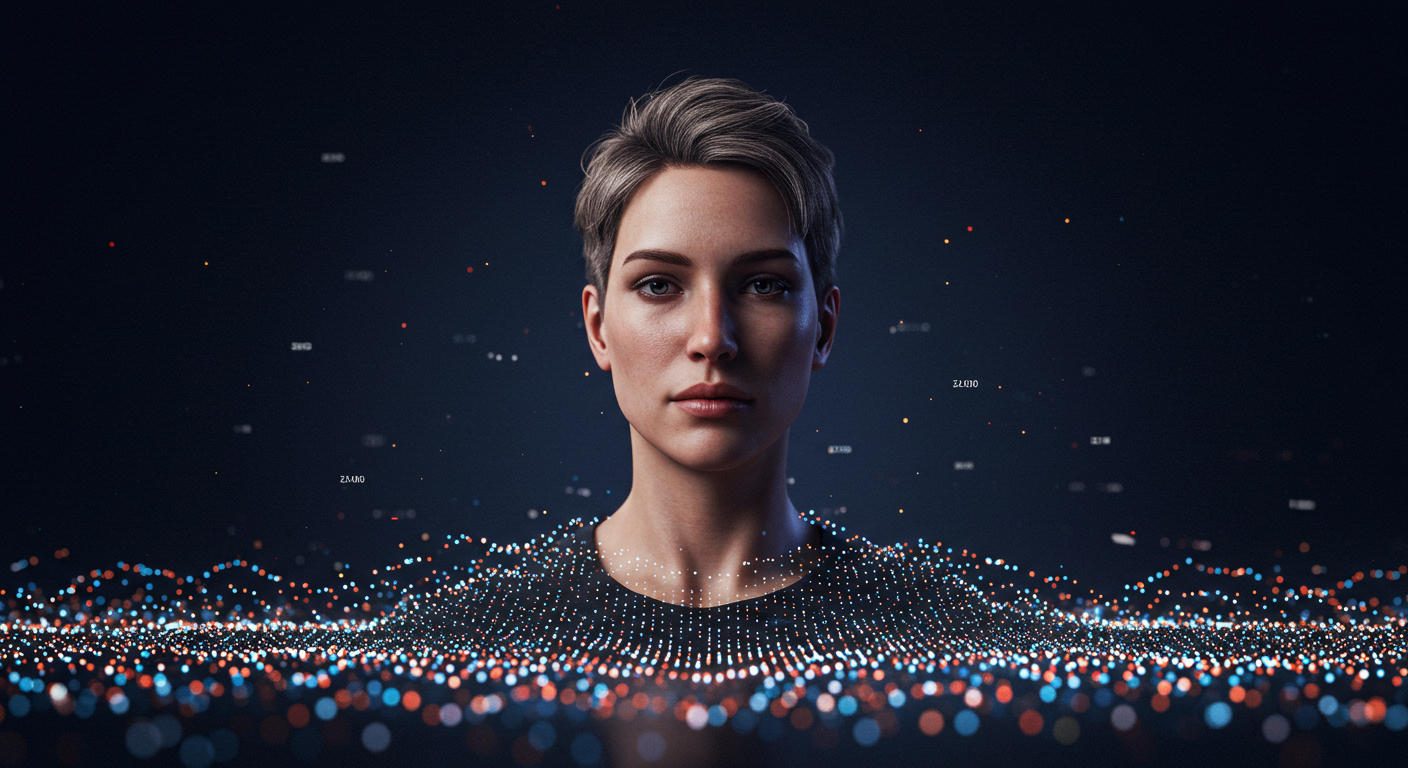
Q0 AI: The Behavioral Intelligence Layer for the Digital Workforce
Project team
David van Dijk, Assistant Professor of Medicine and Computer Science
Olin Geimer, Postgraduate Fellow
Ivan Vrkic, Postgraduate Associate
Q0 AI provides the intelligence layer for a digital workforce—teams of AI agents—that collect, manage, and analyze human-generated data. Every organization depends on human behavior, making a deep understanding of these patterns essential for success. Our advanced behavioral foundation models and intelligent analytics tools seamlessly integrate into solutions powered by teams of AI agents, enabling organizations across business, policy, marketing, and healthcare to optimize services, drive value, and effectively leverage the core behavioral patterns.
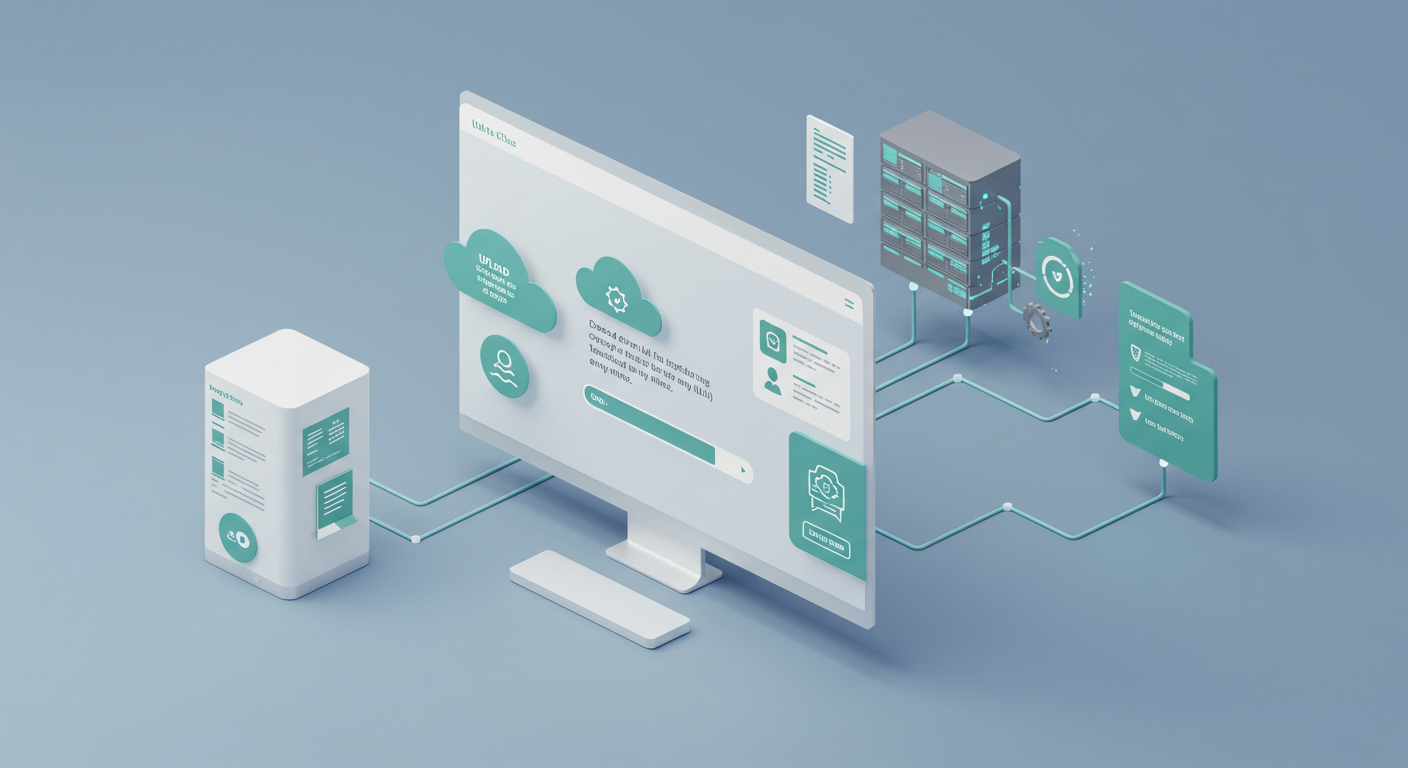
ExpBuddy: Domain expertise agent in the cloud via specialized large language models
Project team
Rex Ying, Assistant Professor of Computer Science
Ali Maatouk, Postdoctoral Associate
Despite their success, Large Language Models (LLMs) lack specialization, as they focus on knowledge from diverse domains. This lack of specialization hinders their performance compared to domain-specific LLMs. The team is working to create a unified cloud service to develop specialized LLMs for any domain of interest through a simple interaction with a web-client interface. Leveraging their AI product, users can create domain-expert LLMs for any field, no matter how specific, through a process that is transparent and accessible to the user.
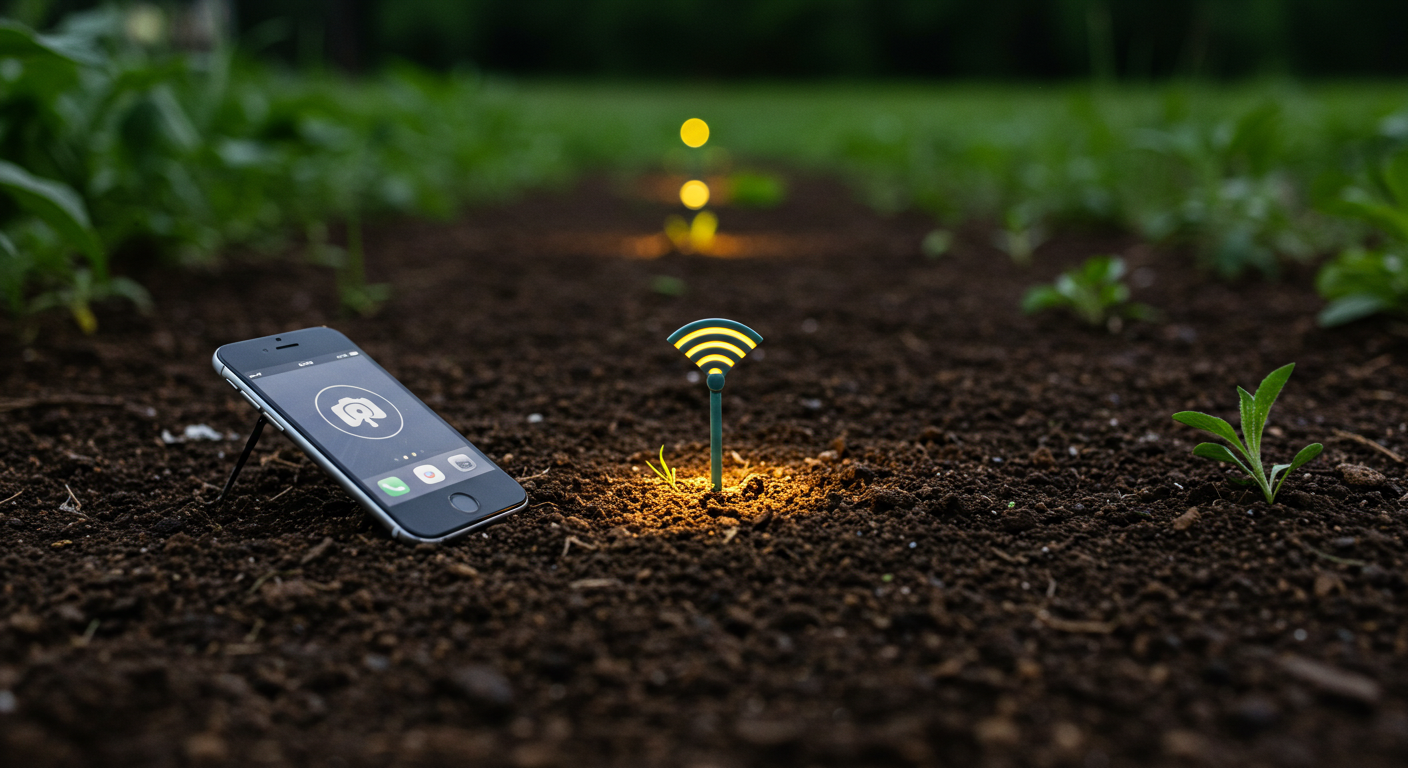
Low-cost soil sensing with Wi-Fi and machine learning
Project team
Leandros Tassiulas, John C. Malone Professor of Electrical & Computer Engineering & Computer Science
Jian Ding, Ph.D. candidate
Accurate, inexpensive, and rapid determination of soil properties is an essential requirement for data-driven agriculture. In this project, the team has focused on using widely accessible Wi-Fi hardware and smartphone images to measure soil moisture, electrical conductivity, and carbon content—three essential properties for monitoring soil health and mitigating climate change.
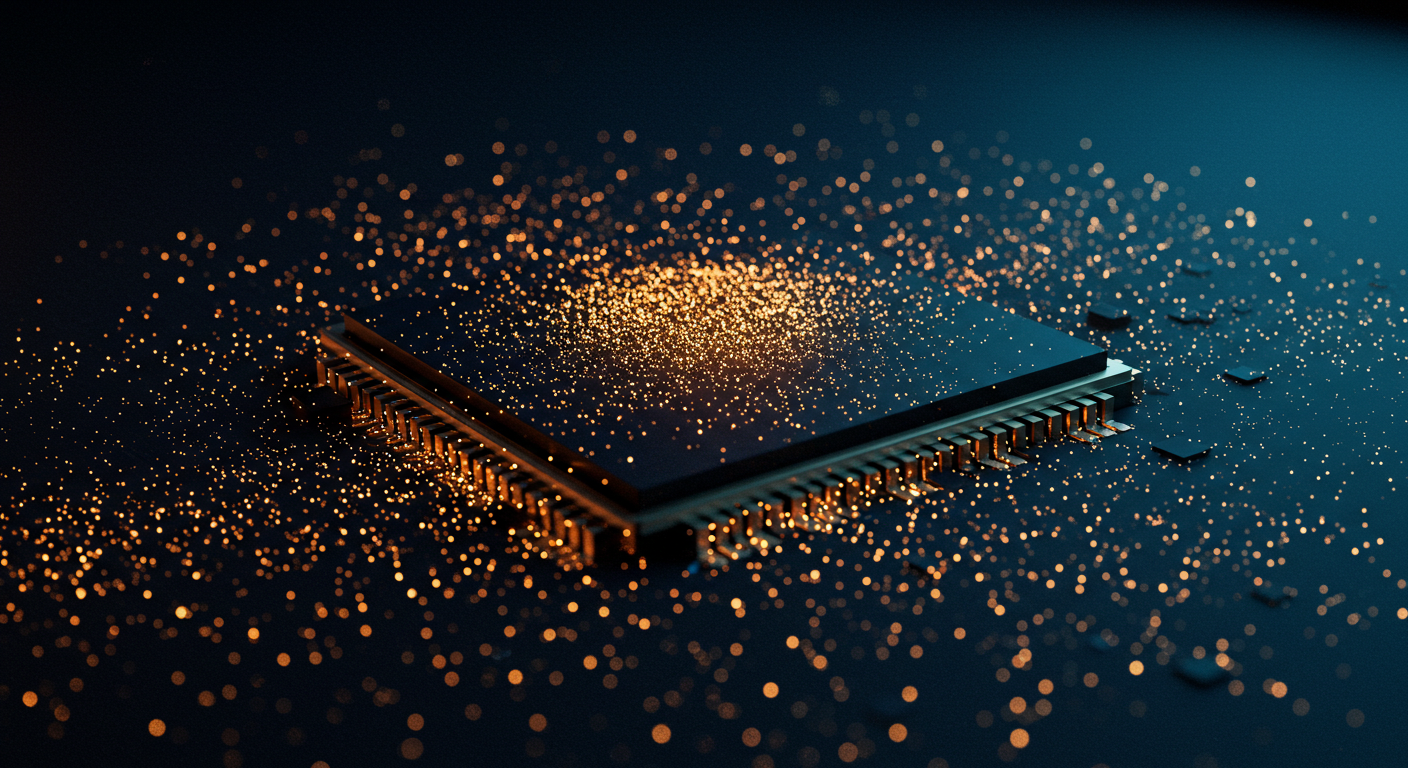
Millivolt AI: Ultra-low voltage circuits for energy-efficient AI
Project team
Logan Wright, Assistant Professor of Applied Physics
Jinchen Zhao, Ph.D. candidate
Jared Wyetzner, Yale College'26 (Physics)
Millivolt AI aims to revolutionize AI hardware by enabling inference and training to use up to a million times less energy. Practically, their technology is in many ways a software upgrade that relies on deep insight into hardware physics. Their vision is to power large-scale sustainable AI-development.
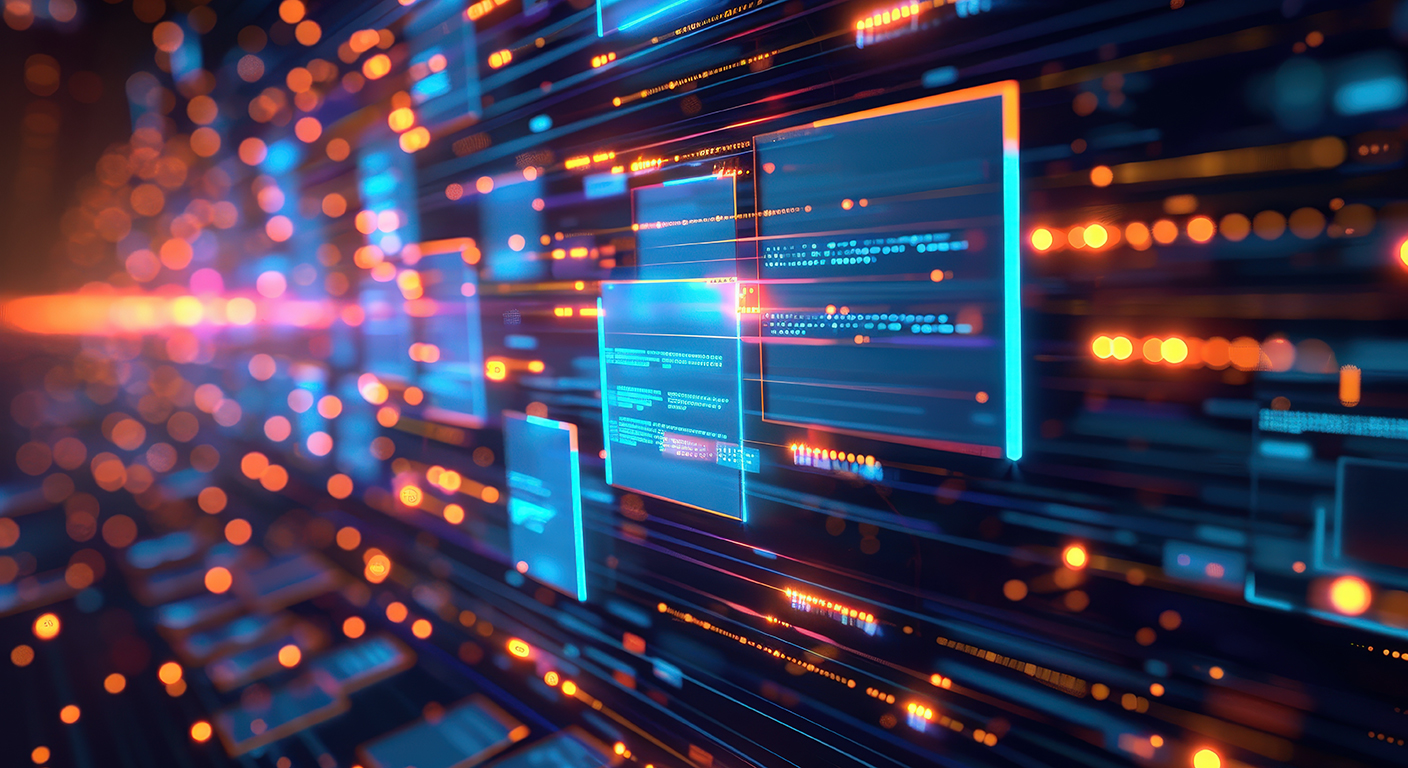
PARTEE: Rich I/O and availability for safety-critical, privacy-sensitive robotics and IoT software
Project team
Zhong Shao, Thomas L. Kempner Professor of Computer Science
Richard Habeeb, Ph.D. candidate
PARTEE aims to protect the most safety-critical software tasks running on modern robotics while also providing access to complex, rich I/O. The team is working to combine a few major technologies: a partitioning system which isolates critical software, a secure inter-task communication protocol, and a mechanism to send and receive complex I/O.
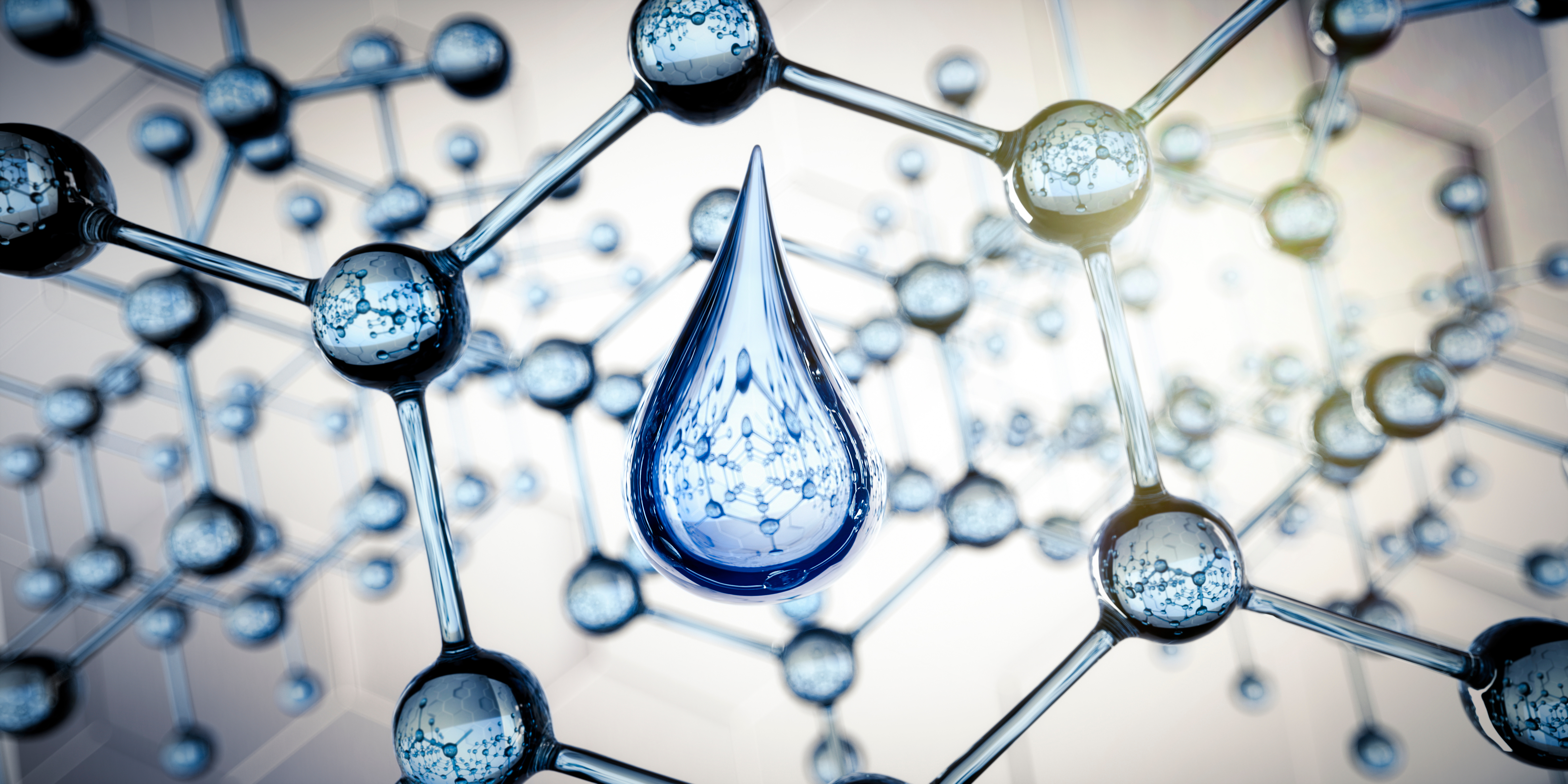
Photochemical carbon removal from seawater
Project team
David Kwabi, Associate Professor Chemical & Environmental Engineering
Byron Ross, Incoming Postdoctoral Fellow
Bin Yun, Ph.D. candidate
Ava Canney, Yale College ’26 (Chemical Engineering)
The Kwabi Lab has pioneered a groundbreaking approach to carbon mitigation by leveraging the ocean’s natural ability to absorb CO₂ from the atmosphere. Traditional photochemical and electrochemical systems for seawater carbon removal are often complex and energy-intensive, limiting their scalability and widespread adoption. By harnessing light-driven carbon removal, the team is reimagining carbon capture at scale, offering a next-generation solution to one of the world’s most pressing climate challenges.
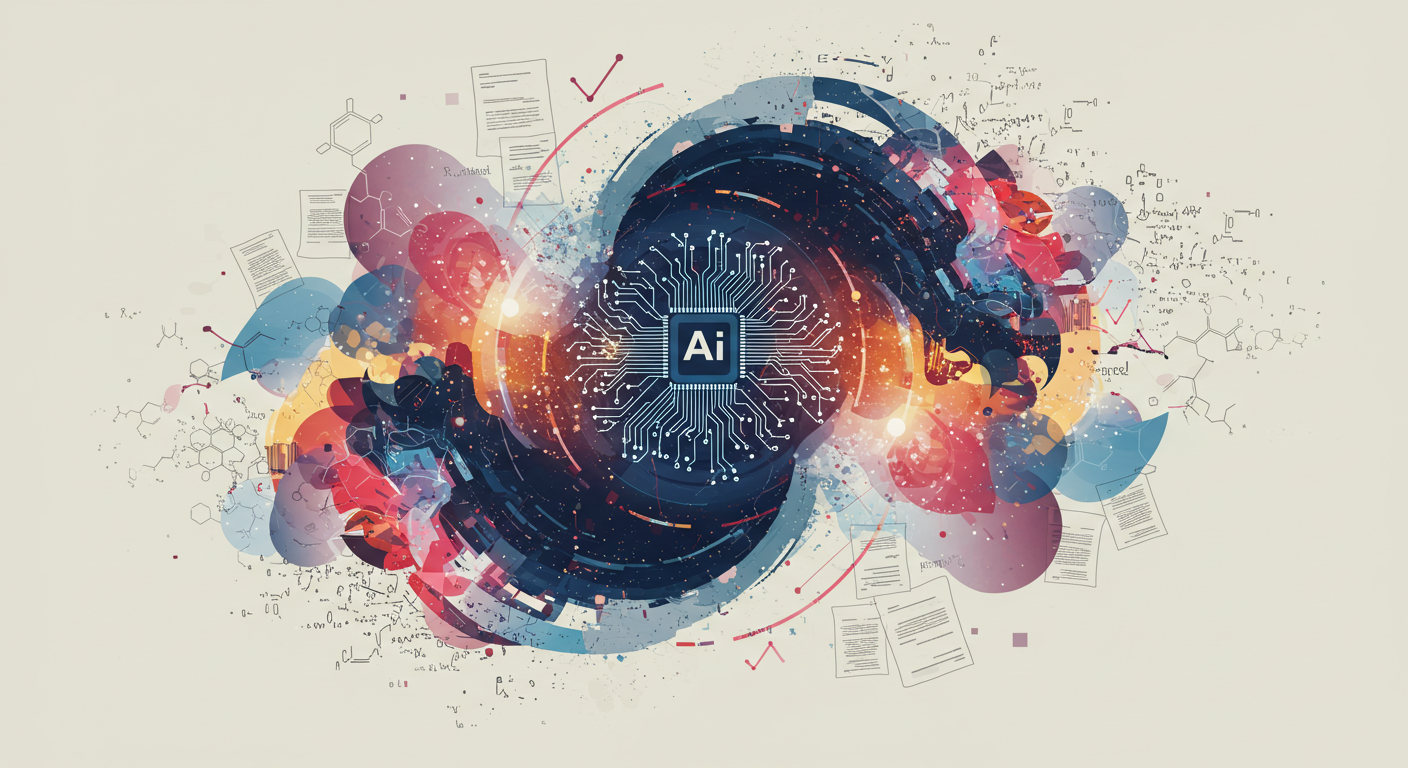
SciMentor: LLM agents as research advisors
Project team
Arman Cohan, Assistant Professor of Computer Science
Yilun Zhao, Ph.D. candidate
Alan Li, Ph.D. candidate
SciMentor is an AI-powered platform that serves as a virtual research advisor for early-stage researchers. SciMentor leverages advanced Large Language Models (LLMs) within an innovative LLM-Agent framework to provide adaptive, iterative mentorship, mirroring the guidance of domain-specific experts. It addresses key challenges faced by junior researchers, including limited access to mentorship, time-consuming literature reviews, and the need for rapid hypothesis validation.