Innovator Spotlight: Dr. Gigi Galiana on making AI work for radiologists through innovation and entrepreneurship
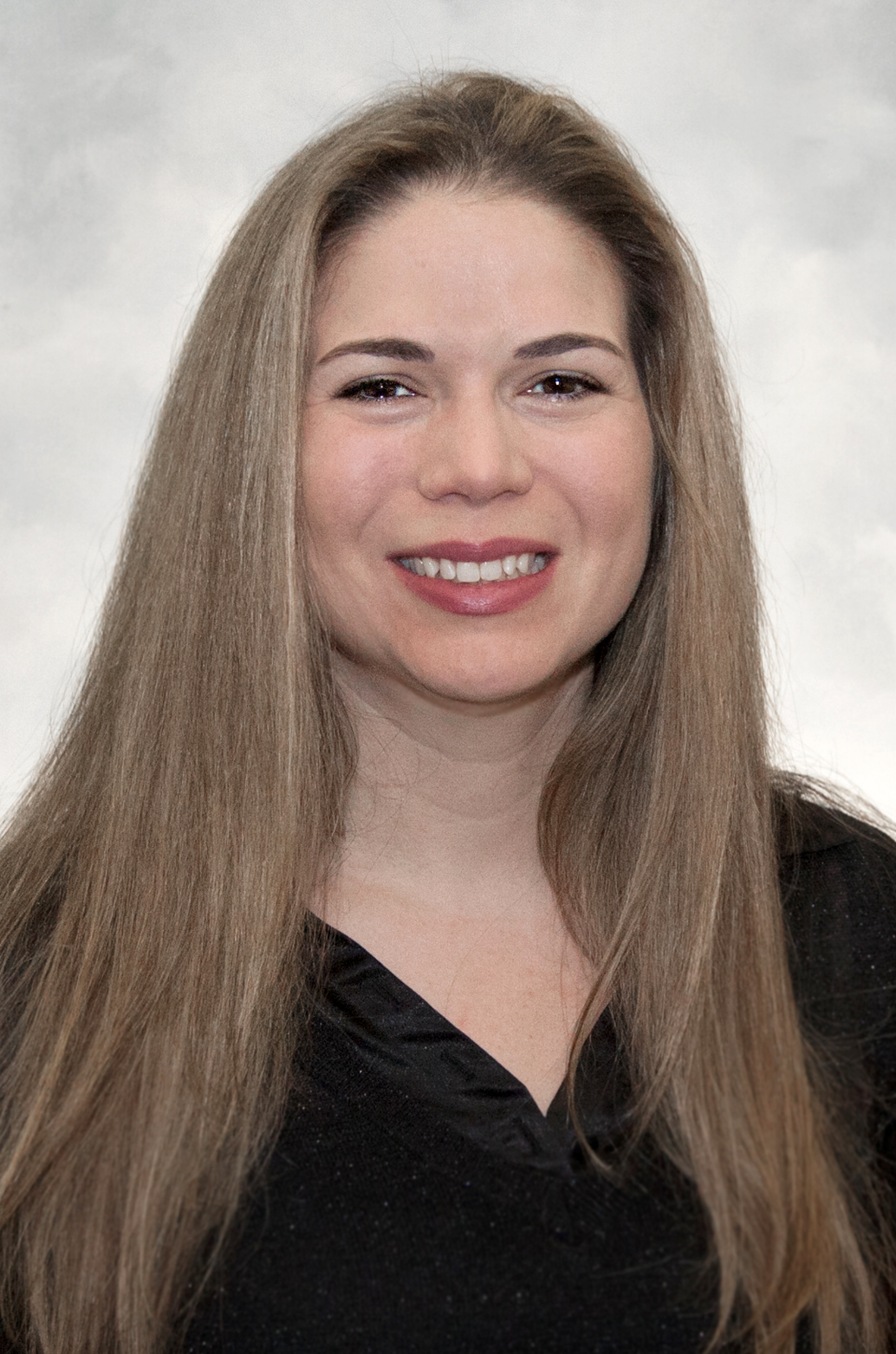
My general impression is that investor-funded startups are trending more toward risky, basic science work that can be really high-impact. I think it's really great that Yale and Yale Engineering are embracing this culture, and encouraging faculty to make use of these new opportunities that are opening up.
Dr. Gigi Galiana is Associate Professor of Radiology and Biomedical Imaging (Yale School of Medicine), and Biomedical Engineering (Yale School of Engineering & Applied Science), and a recipient of a Roberts Innovation Fund award. An MRI physicist, Dr. Galiana is an expert in Magnetic Resonance physics and chemistry. In addition to her research, Dr. Galiana is an entrepreneur and the co-founder of e-CAMP, a physics-based algorithm to standardize MRI for AI.
#1 Please provide a very brief overview of how MRI imaging works.
MRI is an imaging modality, and MRI signals come from detecting the nuclear moments of spins: in humans, water is the main source of detectable spin. So, let's just say it detects water; an MRI is basically a graph of where water is.
What's really interesting about MRI is that if it only made plots of how much water is in your body, it would not be a very powerful modality. With MRIs, we can get something called weighted images. So, for example, you can get an image that's density-weighted, and that is just a plot of how much water is in each pixel of some slice in your body. You can run different kinds of scans too though - for example, you could run a diffusion weighted scan. In a diffusion weighted scan, if the water molecule is diffusing rapidly, it'll go dark, and if it's diffusing slowly, it'll be bright. So, MRI is mostly about imaging water, but we have many ways to weight the signal based on microstructural characteristics, like how quickly the water is diffusing, how big the molecule is, and how quickly it’s tumbling. We can run different experiments that amplify those effects, giving the image different contrasts that highlight different features.
Let's take the case of cancer: water content in healthy tissue versus a tumor is very similar. If you take a density-weighted image, it would mostly just tell you there's tissue there. But, if you take a diffusion-weighted image, then the cancer comes out bright, and the regular tissue is dark, because at the microstructure level, cancer doesn't have those big, fluffy, balloon-like cell structures that healthy tissue does. Things are more collapsed and the water is more constrained, so it doesn't diffuse as freely. A diffusion weighted image gives us the contrast that informs doctors of which tissue is healthy and which is cancerous.
A T2-weighted image is a contrast acquired in almost every single clinical MRI scan. It gives a lot of different useful contrast about water, potential tumors, structural resolution, and it's pretty fast. So, people just toss it in there as a standard sequence in almost every kind of workup of different clinical questions for different organs.
#2 What is qMRI?
Quantitative MRI (qMRI) is not clinically common. It's certainly done, probably in every hospital, but I think it's fair to say that volume-wise, it's a tiny percentage of clinical MRI. With quantitative imaging, you're not trying to get an image that reflects a certain weighting, you're actually trying to get the number. For example, with diffusion imaging, you're trying to get enough data to calculate the diffusivity in that pixel in quantitative units, such as millimeters squared per second (mm²/s).
With clinical MRI, there are a lot of dials that need to be turned, and each hospital will choose different settings on those dials. For example, I can get a standard weighted image in hospital A and the tumor may be 2x brighter than the healthy tissue, while in hospital B, the tumor might be 5x brighter, all because of those different tuning parameters. On the other hand, a qMRI will give you the same number in both hospitals.
Here’s one analogy: let’s say you have 200 pictures of dogs, and they’re all taken with different cameras, and different lightings - maybe there's some filter that can make them all look as if they were taken at noon, on a beach, in New Haven. There are people doing better and better jobs with those filters. There's a similar thing that you can try to do with MRI images - take the weighted images, and normalize them in different ways, but, at the end of the day, it's going to be very hard to say whether or not one dog's coat is really darker than another dog's coat after the pictures have been through those filters and complex manipulations.
A qMRI would be like taking an absorption spectra of the dogs coats. That way, it doesn't depend at all on how the lighting was - you're really getting to the fundamental character of the color. With qMRI, you're getting a quantitative measure of the fundamental physical property of the water spins that you're looking at.
#3. Your technology, e-CAMP, calculates qMRI from a regular, routine MRI scan using AI technology. Please talk about how your technology works, its applications, and potential for impact.
Background:
e-Camp is something I developed with Hemant Tagare. It was a joint idea that evolved between us. Hemant’s background is more in math and algorithms - he's an image processing expert. I'm more of a physicist and experimentalist, and I also work on different hardware projects.
We came up with a method to generate qMRI even from very limited data. We set for ourselves the challenge of, ‘what if the data we had was just the data from the standard weighted image, but we treat it as if it was a very poorly planned qMRI experiment, and calculate the qMRI from that data that’s acquired routinely.’ And, it worked! We were able to get quantitative images that were quite accurate even from routine weighted images.
Problem: A lack of training data to advance AI for radiologists
What we felt is powerful about e-CAMP is that people are increasingly trying to develop AI to triage MRI images and guide radiologists in terms of where to shift their attention when analyzing scans. But, one of the challenges is that people are taking a training set of MRI scans from one hospital, let's say from one or two sites, they train their data and get very high accuracy, but when they take that AI and apply it to a different hospital, the performance always goes significantly down because of those tuning parameters that I was describing before in the weighted images.
People are aware of this problem, and they try and fix it the way you would with a photographic filter: send it through something, match the intensities, match the brightnesses, match the histograms, etc. But, when you do that, you're losing something about the information content in the original image. We thought that if all the training was based on qMRI, you could just eliminate this problem.
A complaint that we hear from a lot of our clinical colleagues is that, currently, a lot of the AI tends to point out the obvious and and miss the things that are hard for radiologists to see. If we really want AI to help radiologists, it needs to see beyond what radiologists can, or see things that they can barely see (finer and finer biomarkers) - that's where these photographic filters can really make it difficult to pull out those details.
Solution & Potential for Impact:
We thought of working with qMRI since it has all the relevant clinical parameters. The idea is that we can take the data that's routinely acquired and pull out the qMRI that should be the same from site to site, instead of the regular weighted image that has so much variability in different hospitals.We thought that this could be a really powerful training source for AI. 40 million scans are conducted per year, almost all of which are going to include a standard T2 weighted image. If you could turn all of them into quantitative T2 images, it would be a massive, massive source of AI training data.
Learn more about AI training here.
Our project has to do with creating better AI and, more specifically, creating more generalizable AI. There's a shortage of radiologists. There are big and very promising efforts to actually create more MRIs. On the hardware side, there are a lot of efforts to make MRI more accessible and cheaper. So, I think that the impact of AI-assisted radiology could be very high.
An unmet need:
We have not found a study that trains an AI strictly on quantitative images, which makes sense because it's hard to pull together a large number of quantitative images. The closest thing is a prostate MRI. There's one kind of quantitative image that is routinely done, and the whole exam is basically two images, one quantitative and one regular weighted image. There is evidence in the literature that the weighted image is the weak link. When they try to train AI and each person has two images, the quantitative image carries more information than the non-quantitative image, pulled across many different scans on different scanners, despite the fact that radiologists find the weighted image very important. We think that the variability makes it difficult. We haven't seen studies comparing AI on quantitative versions of an image versus non-quantitative versions of an image, across numerous sites. That's something that we want to demonstrate with our Roberts Award - that's our milestone.
#4 You are a Roberts Innovation Fund Awardee! Dean Jeffery Brock said "We have always had the ideas at Yale Engineering; we were lacking the infrastructure needed to make this leap." Have you noticed a culture shift for researchers to not only conduct important research, but also ponder how it can be most transformational?
I think the Roberts Innovation Fund is a very wonderful initiative. In general, I’m impressed with the resources, knowledge and willingness to support researchers at Yale Engineering, and provide researchers with the opportunities to pursue impactful projects.
I do think that there's been a culture shift in encouraging faculty to engage more with what's happening in the startup side of the world. My general impression is that investor-funded startups are trending more toward risky, basic science work that can be really high-impact. I think it's really great that Yale and Yale Engineering are embracing this culture, and encouraging faculty to make use of these new opportunities that are opening up. Yale has so many resources and there are so many great ideas around here, so I’m very hopeful for these kinds of initiatives.
#5 Can you share more about your experience taking the leap from idea/research to the pursuit of commercialization for optimal impact on society? How have you used the Roberts Innovation Award to advance your technology?
The Roberts Innovation Fund Award came with funding, which helped power us through some new goals, but it has also come with a great deal of training. Claudia Reuter, Director of The Roberts Innovation Fund, has been tremendously helpful in getting us all sorts of coaching and brainstorming opportunities to help us understand that shift in mindset needed to make your work widely understandable. Academics get comfortable speaking to other academics - taking a step back and speaking a bit more ‘bigger picture’ is not something we do everyday, so it’s been very helpful to improve those skills.
#6 As a woman in both academia and innovation, can you describe some of the challenges you have faced, how you have overcome them/are overcoming them?
I’ve been quite fortunate to have excellent mentors and support. Most of my senior mentors have been men, because that’s the makeup of my field, but support is support. We shouldn’t think that women only need women to encourage them. We need leaders who believe in us - anyone you respect and admire can be a great mentor. Nonetheless, there are also increasingly so many prestigious women, both among my colleagues and our leadership, for me to look up to. There has been a lot of progress in our field, and more broadly, the culture has slowly changed, brick by brick. We have many great female role models here at Yale, and I work with a number of excellent female professors within my department whom I go to regularly for advice. So I do think that with the support, programs, and increasing awareness, things are getting better.
#7 What is your best advice for other women interested in entrepreneurship?
For women at Yale, there are excellent and very positive coaching opportunities here, and you don’t have to have everything figured out before you talk to someone. When I first applied to this program, I was intimidated because we did not have a developed business plan. But, people are there to help you work through that. We actually modified our project as part of the application process from the feedback we received from Yale Ventures and some others in the field. Don’t be afraid to just start a conversation - the worst that can happen is they say ‘let’s pause, and come back to it in a few months,’ and that’s ok!
-----------------------------------------------------------------------------------------------------------
This interview was written and compiled by Sonia Seth, Yale SOM ’25, a Yale Ventures summer associate.